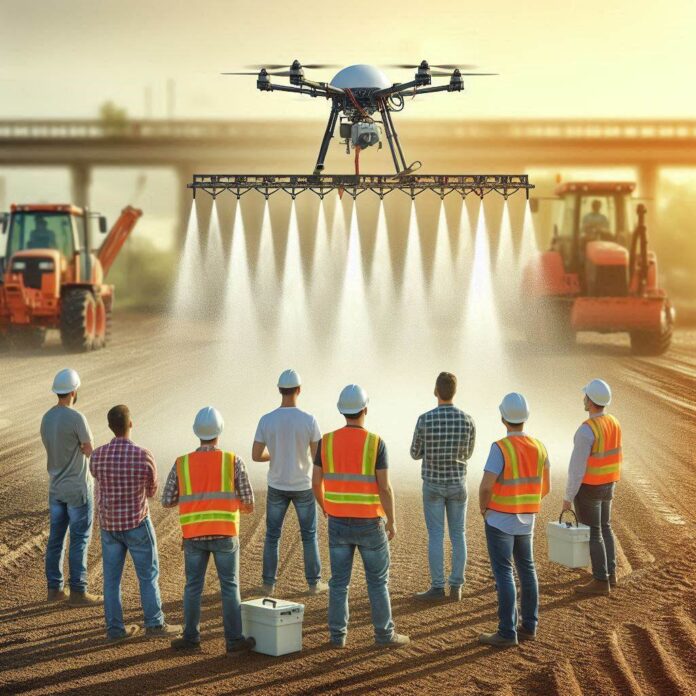
While still a relatively new technology, custom generative artificial intelligence (AI) is now being explored for developing stormwater and other compliance documents, or at least acting as an assistive tool. Consider an AI model trained in all current state-specific construction general permits and consider what prompts, or questions, might be asked to generate the necessary documents. Now, let’s take a deeper dive into this application and examine its future potential within the industry.
Although the end user would need to confirm that the source data is up to date and hasn’t been superseded by a new general permit, the AI could respond to questions like “What are the stabilization requirements in Texas?” by extracting all relevant references to stabilization directly from the permit. Professionals who have developed a Storm Water Pollution Prevention Plan (SWPPP) for a state with a non-searchable general permit, such as a photocopy on a state website, are familiar with the struggle of repeatedly scanning the permit to avoid missing any crucial detail or exception. This is merely one example of how custom generative AI models can be utilized. Alternatively, they could be trained on various Code of Federal Regulations, state administrative codes, local ordinances and more.
Looking to the future, new AI-powered applications have the potential to enhance SWPPPs and other compliance plan preparation. For instance, machine learning algorithms can analyze historical data to identify patterns and trends to facilitate predictive modeling for potential risks and optimization of BMP selection and mitigation measures, such as BMP maintenance. AI-based programs could potentially prepare SWPPPs and other compliance plans, while natural language processing algorithms could enable efficient data extraction from documents and assist in automating compliance reporting tasks and permit filing. These advancements have the potential to contribute to reducing preparation time while improving overall plan efficiency.
An intriguing prospect arises with the development of agentic AI (Figure 1). Agentic AI consists of AI agents that are designed to act independently of human intervention, unlike traditional AI that depends on user inputs, to understand their environment, set goals and act to achieve those goals.1 Imagine the possibility of an AI agent trained to launch scheduled drone flights to collect data, analyze patterns in that data, generate predictive modeling and initiate preventive actions such as robotic machines to repair silt fence, mow vegetation, etc. While this may sound like something from a futuristic sci-fi movie, leading companies such as Tesla and Nvidia, along with smaller startups, are actively working to turn these ideas into reality.
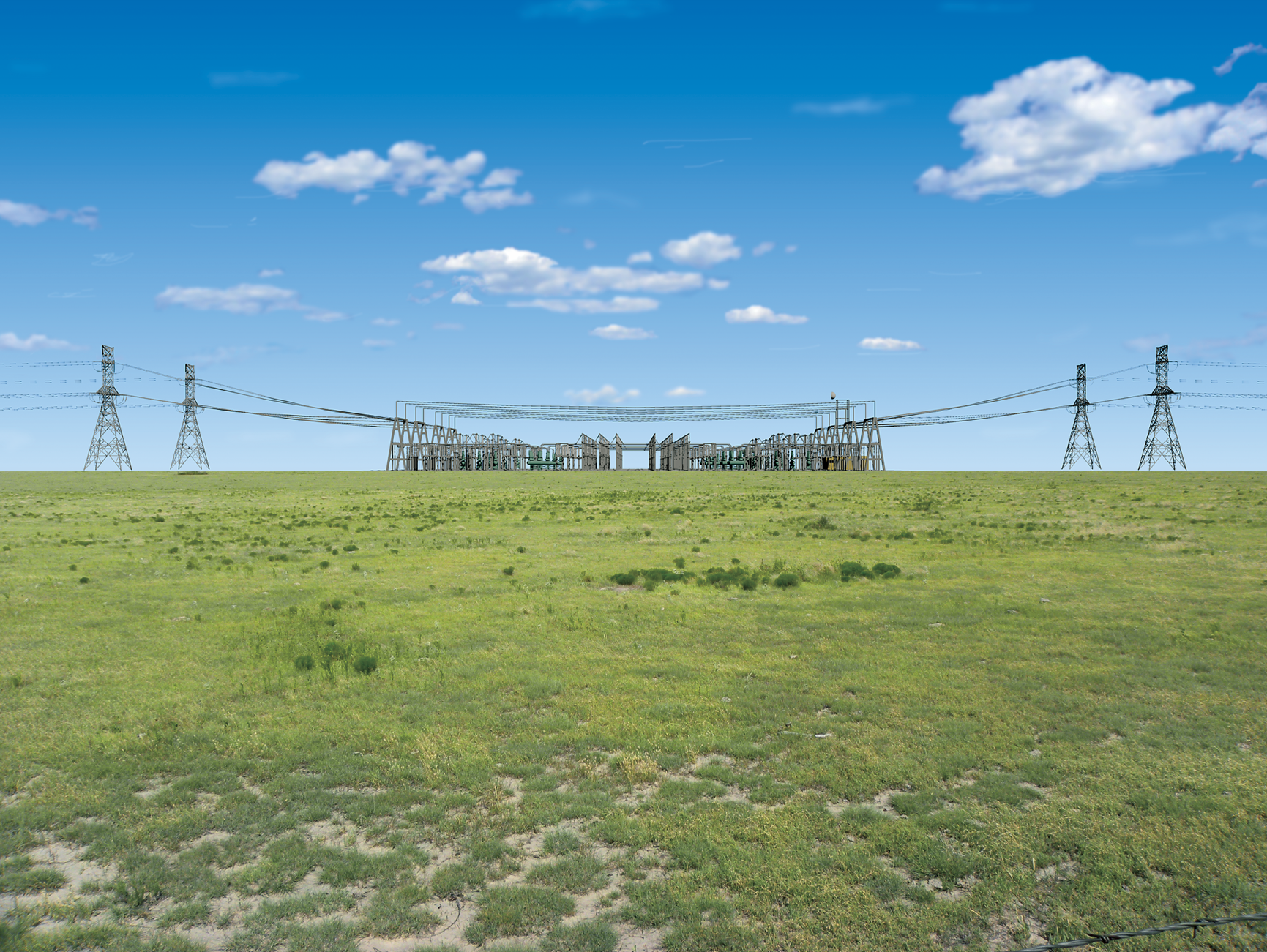
Navigating the Risks
While AI is advancing rapidly, its widespread adoption still faces significant challenges. One of the primary concerns is the current state of the technology itself. Despite notable progress, AI systems remain imperfect, as users can attest. Many AI applications, such as autonomous systems, still struggle with unpredictable real-world scenarios like self-driving cars, which perform well in controlled conditions but fail to handle complex, unforeseen events. Factors that can contribute to the challenges of the current state of AI technology include limited data sets or erroneous/outdated source data, trainer bias in the model and user error.
As the excitement and enthusiasm of the initial onset of generative AI begin to fade, there is a shift as the reality of AI use sets in. Companies are struggling to answer the question of how AI can be integrated into their existing systems and workflows, and that includes those in the environmental compliance industry. Users are quickly learning that having vague concepts of an idea for use makes it difficult to build out generative AI models. An example of a vague concept would be “Let’s build an AI that generates SWPPPs.” It is important to have a very detailed, clearly defined use case.1
The adaptation and integration of AI-powered tools in compliance documentation compliance also introduces inherent risks in the form of significant legal and ethical concerns. AI models must be designed to navigate a complex web of legal considerations, ranging from data privacy laws and confidentiality agreements to intellectual property rights and liability issues regarding compliance with industry-specific regulations. Moreover, the user must also be aware of this necessity.
Additionally, there are growing concerns about transparency and accountability in AI decision-making processes. As AI models become more complex, the “black box” nature of some systems makes it difficult to audit or fully understand how decisions are being made, which poses a risk regarding regulatory compliance and ethical governance. Because AI systems are trained on historical data, they can inadvertently learn and perpetuate existing biases present in that data. AI systems often rely on vast datasets to function effectively, and some of this data comes from published intellectual property collected through digital platforms. Use of this data raises questions about consent, ownership and how that data is stored, shared and analyzed.2
These risks highlight the importance for regulation in the industry that calls for appropriately vetting the data and algorithms on which the AI is trained and transparency by developers. Ethical frameworks and guidelines such as data anonymization and bias reduction techniques will be essential to foster trust.2 These risks are becoming highly recognized as evidenced by the world’s first comprehensive AI law, the European Union’s AI Act, which took effect August 2024.
Another potential future challenge in the development and advancement of AI lies in the continuing efforts for greener infrastructure for the data centers that AI compute relies on, particularly when considering the massive infrastructure required to support it. According to the United States Office of Energy Efficiency and Renewable Energy, these facilities are among the “most energy-intensive building types.”3 (Figure 2) These facilities also use a considerable amount of water in cooling systems. According to NPR, their “reliance on water poses a growing risk to data centers.”4 This usage consumption scale raises critical concerns about the environmental impact of AI development and the related strain on local infrastructure. Balancing the growth of AI capabilities with environmental responsibility is becoming an urgent consideration as the industry moves forward at an ever-accelerating pace.
The future of AI holds immense potential for innovation and efficiency in development of stormwater and environmental compliance documentation. As custom-built models and advanced systems become more prevalent, it is crucial for organizations to address the associated risks and ethical considerations proactively. Ensuring the continuous improvement of data quality, promoting transparency in AI decision-making and adhering to strong legal and regulatory frameworks will be key to navigating this rapidly evolving field. By aligning the transformational potential of AI with socially responsible and ethical practices, organizations can effectively leverage these technological advancements to enhance efficiency and quality in stormwater and environmental compliance document development.
Editor’s Note: The first part of this series appeared in the First Quarter 2025 issue of Environmental Connection and reviewed
the emergence of the AI revolution, today’s capabilities and current applications.
References
- Craig, L. (2024) 10 top AI and Machine Learning Trends for 2024: TechTarget, Enterprise AI. Available at: https://www.techtarget.com/searchenterpriseai/tip/9-top-AI-and-machine-learning-trends (Accessed: February 2024).
Patel, K. (2024) ‘Ethical Reflections on Data-Centric AI: Balancing Benefits and Risks’, International Journal of Artificial Intelligence Research and Development (IJAIRD), 2(1), pp. 1–17. - Data Centers and Servers (no date) energy.gov. Available at: https://www.energy.gov/eere/buildings/data-centers-and-servers (Accessed: 2024).
- Copley, M. (2022) Data Centers, backbone of the digital economy, face water scarcity and climate risk, NPR. Available at: https://www.npr.org/2022/08/30/11199
38708/data-centers-backbone-of-the-digital-economy-face-water-scarcity-and-climate-ris (Accessed: 2024).
About the Experts
- John England is a lead environmental scientist for Black & Veatch’s Construction Stormwater and Environmental Compliance practice. He provides environmental support and input during proposal and project development, including implementing and managing overall environmental compliance efforts during construction.
- Kayla Cottingham leads the Construction Stormwater and Environmental Compliance practice at Black & Veatch. She leads the team’s environmental support and input during proposal and project development, including implementing and managing overall environmental compliance efforts during construction.